
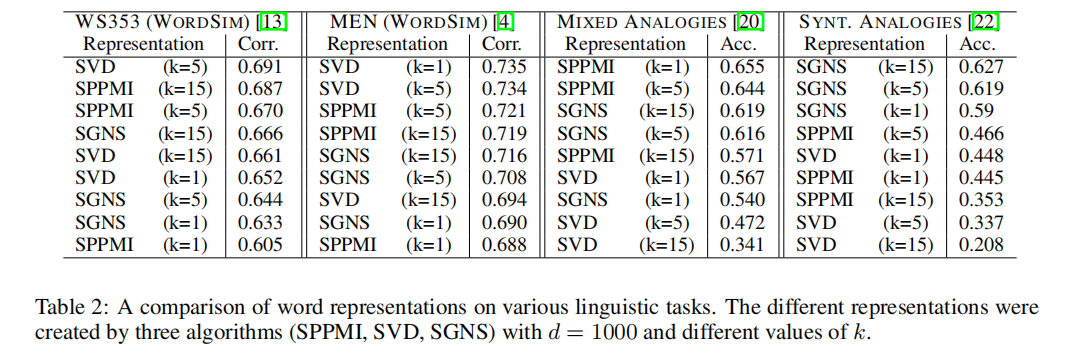
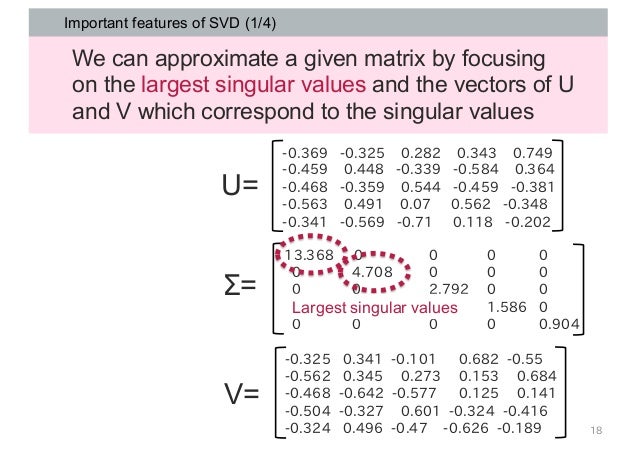
On analogy questions SGNS remains superior to SVD. When dense low-dimensional vectors are preferred, exact factorization with SVD can achieve solutions that are at least as good as SGNS's solutions for word similarity tasks. This paper draws both empirical and theoretical parallels between the embedding and alignment literature, and suggests that adding additional sources of information, which go beyond the traditional signal of bilingual sentence-aligned corpora, may substantially improve cross-lingual word embeddings. The relationships among various nonnegative matrix factorization methods for clustering ICDM06. Omer Levy, Anders Sgaard, and Yoav Goldberg. We show that using a sparse Shifted Positive PMI word-context matrix to represent words improves results on two word similarity tasks and one of two analogy tasks. Neural word embedding as implicit matrix factorization NIPS. In Proceedings of the 27th International Conference on Neural Information Processing Systems. Both approaches: Rely on the same linguistic theory Use the same data Are mathematically related Neural Word Embedding as Implicit Matrix Factorization (NIPS.
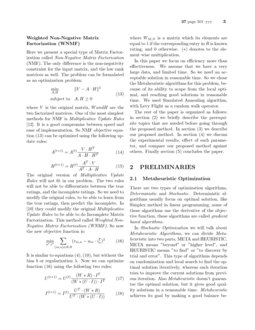
We find that another embedding method, NCE, is implicitly factorizing a similar matrix, where each cell is the (shifted) log conditional probability of a word given its context. Neural word embedding as implicit matrix factorization. We analyze skip-gram with negative-sampling (SGNS), a word embedding method introduced by Mikolov et al., and show that it is implicitly factorizing a word-context matrix, whose cells are the pointwise mutual information (PMI) of the respective word and context pairs, shifted by a global constant. matrix factorization for interactive topic modeling and document clustering.
